Program
- 9:00 - Registration
- 9:15 - Welcome
- 9:20 - Keynote Talk: Prof. Josep Domingo-Ferrer (URV, Serra Húnter Program): The Serra Húnter Programme for faculty excellence
- 10:10 - Session 1
- 11:00 - Coffee Break
- 11:30 - Session 2
- 13:10 - Lunch
- 14:30 - Ceremony of 10th Anniversary of the PhD program
- 15:10 - Keynote Talk: Dr. Sara Hajian (Eurecat): Detecting Algorithmic Discrimination
- 16:00 - Coffee Break
- 16:30 - Session 3
- 17:40 - Closure
You can download the complete program here
Invited Talks
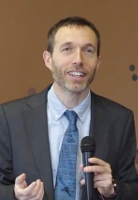
TITLE:
The Serra Húnter Programme for faculty excellence.
SPEAKER:
Josep Domingo-Ferrer
Academic Director
Serra Húnter Programme
ABSTRACT:
The Serra Húnter Programme (SHP) promotes the hiring of highly qualified faculty members with academic records meeting high-level international standards. The SHP is part of a new academic staff model that the Government of Catalonia is promoting to reinforce the internationalization of the Catalan universities, with the ultimate goal of consolidating Catalonia as the knowledge hub of Southern Europe. Positions are offered in the ranks of non-tenured assistant professor, tenured associate professor and tenured full professor. Successful candidates will be hired by a Catalan university, and they are expected to cooperate with existing research groups or to develop new lines of research, complementary to those already in place. This talk will describe the selection process and the career expectations of Serra Húnter faculty members.
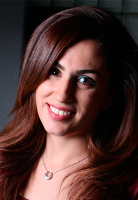
TITLE:
Detecting Algorithmic Discrimination.
SPEAKER:
Sara Hajian
Research scientist
Eurecat Technology Center
ABSTRACT:
Algorithms and decision making based on Big Data have become pervasive in all aspects of our daily (offline and online) lives, as they have become essential tools in personal finance, health care, hiring, housing, education, and policies. Data and algorithms determine the media we consume, the stories we read, the people we meet, the places we visit, but also whether we get a job, or whether our loan request is approved. It is therefore of societal and ethical importance to ask whether these algorithms can be discriminative on grounds, such as gender, ethnicity, marital or health status. It turns out that the answer is positive: for instance, recent studies have shown that Google’s online advertising system displayed ads for high-income jobs to men much more often than it did to women.
This algorithmic bias exists even when there is no discrimination intention in the developer of the algorithm. Sometimes it may be inherent to the data sources used (software making decisions based on data can reflect, or even amplify, the results of historical discrimination), but even when the sensitive attributes have been suppressed from the input, a well trained machine learning algorithm may still discriminate on the basis of such sensitive attributes because of correlations existing in the data.
From technical point of view, efforts at fighting algorithmic bias have led to developing two groups of solutions: (1) techniques for discrimination discovery from data and (2) discrimination prevention by means of fairness-aware data mining, develop data mining systems which are discrimination-conscious by-design. In this talk I mainly focus on the first groups of solutions: In the first part, I will present some examples of algorithmic bias, followed by introducing the sources of algorithmic discrimination, legal principles and definitions and finally measures of discrimination applied in fairness-aware data mining solutions. In the second part, I will introduce some of the recent data mining and machine learning approaches for discovering discrimination from the database of historical decision records.